1 Oct 2024
Building a Personal Trainer in Your Pocket: Crafting an AI Fitness App Like Aaptiv
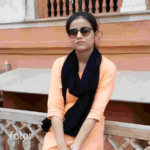
Shifa Akbar
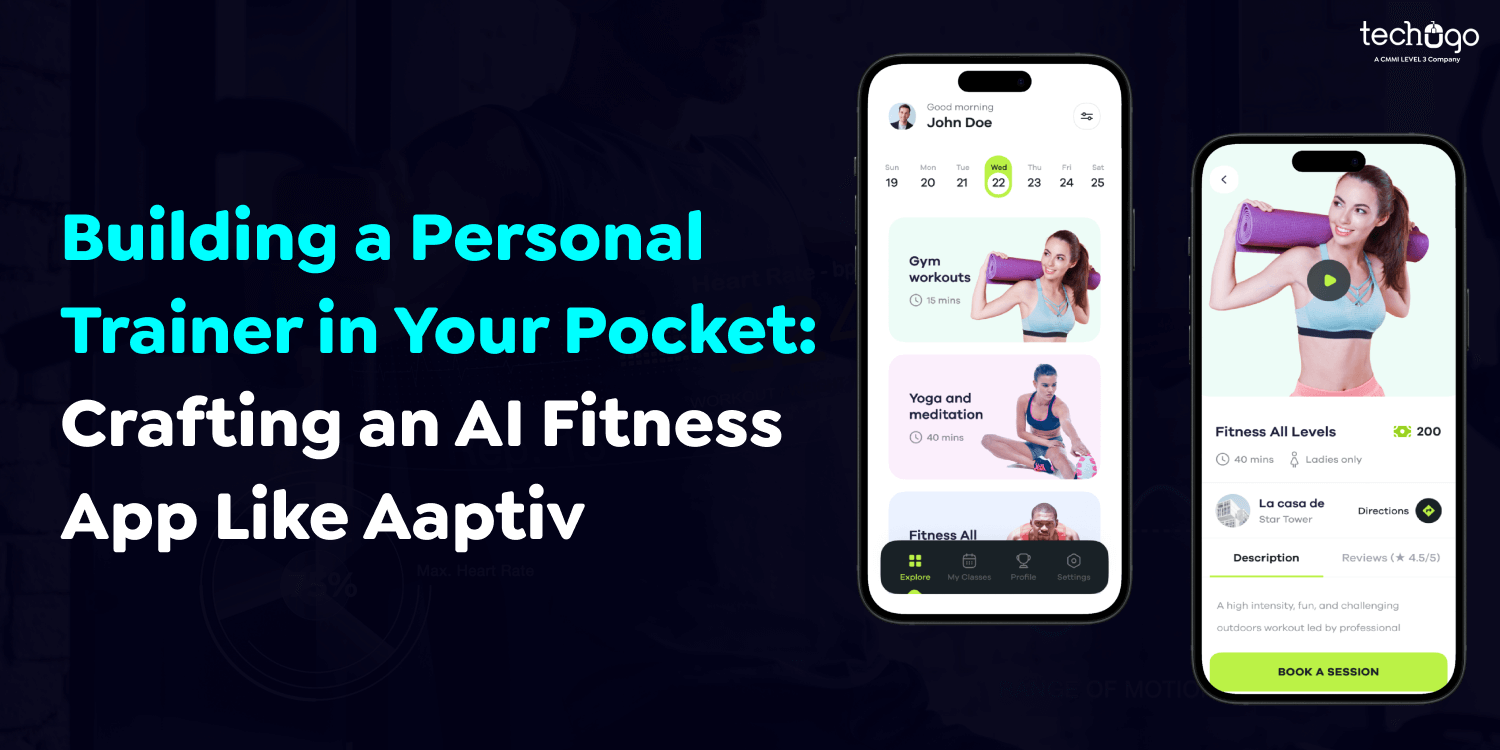
Technological advancements have transformed different sectors, and fitness is no exception. In the past, fitness centers were the leading centers for those looking for private trainers who could help meet the fitness objectives they had set for themselves. But, hiring an individual trainer may be costly for many. This is the point at which AI personal trainer apps can be found.
With the increasing popularity of fitness app development with virtual trainers, the idea of AI personal trainers has gained traction. AI Personal trainers can be described as fitness guides powered by AI for those unfamiliar with the concept. They use user-provided details like body measurements, fitness levels, and objectives to develop individual diet and exercise plans.
The demand for AI-based fitness apps continues to grow, especially those that permit users to exercise from the comfort of their homes. Developing a virtual personal trainer app could be a lucrative business when partnered with a fitness app development company. Before you embark on this path, it is essential to know the inner workings of successful apps like Aaptiv, a top player in the market.
Let us know the most important aspects to consider when creating the perfect AI personal trainer app like Aaptiv, that you must have, including elements, features, technology stack, and many more in the blog. Also, how a fitness app development company serve all these benefits to you. Let’s begin!
Also Read : What All You Require To Make A Successful Fitness App?
What is the Aaptiv App?
Aaptiv is a renowned fitness app with audio and videos designed to offer customized workout experiences. Contrary to other fitness apps, which use text-based instruction, Aaptiv offers workouts guided by trained trainers who are certified through audio-based instruction. It also offers a vast collection of video workouts. Additionally, the app has an array of available exercises that cater to a variety of fitness preferences, including walking, running, and elliptical exercise, as well as stretching, strength training, rowing, yoga, and much more.
The marketplace is full of various breathwork apps, such as meal planning, ad hoc, and others. One of them is Aaptiv. Thanks to AI training, Aaptiv is like a refreshing breath of fresh air. Aaptiv isn’t your typical fitness mobile app. It was launched in 2016 and is now a leading player in the high-end digital health and wellness industry, specifically focused on audio-guided exercises.
Their success is evident by the $50 million in venture capital raised and a loyal customer base that exceeds the 200,000 paid customers. Aaptiv’s impressive track record shows the quality It provides. Aaptiv offers an innovative method of exercise, making high-quality workouts easy and fun, and that’s why it is adored by its users.
Also Read : Must-Have Features For The Health And Fitness Mobile App
AI-Powered Fitness Apps
Here’s a look at real-world fitness apps that use AI to improve fitness results. Let’s meet them and learn from them:
1. Nike Training Club
It’s one of the most effective fitness apps on the market. The app offers a broad selection of free workouts for all fitness levels. Additionally, it employs AI to tailor workouts to users’ goals and preferences.
2. Fitbod
Fitbod is more than just a workout plan application; it’s your trainer with AI in your pocket. This app customizes your workout based on your fitness goals, the availability of equipment, and your previous performances. The system also gives real-time feedback about your form so that you can avoid problems.
3. Aaptiv
Aaptiv is another well-known fitness application. It provides audio-guided workouts that certified fitness trainers guide. The app can also use AI to personalize your workout to match your goals and preferences. The success of Aaptiv highlights the increasing demand for an app similar to Aaptiv that offers AI-powered personalization for fitness professionals.
4. Strava
Strava is an app that is popular for cyclists and runners. It’s a thriving community of athletes competing against each other for greater goals. It uses Artificial Intelligence to monitor your performance over time, detect patterns, and predict your future performance on specific routes. Strava offers the fundamental idea of personalization and community, which is why there is a massive need for more apps that resemble strata development.
5. MyFitnessPal
MyFitnessPal is a cult calorie-tracking application. The app uses AI to monitor your macros and offer individual nutrition guidance. Consequently, it currently has more than 200 million registered users around the world. There is a reason why many people want to develop an app like MyFitnessPal and discover their success on the market.
Also Read : Redefining Wellness in the UK with Innovative Apps : FitTech Breakthrough
5. Peloton
Peloton is a well-known application that provides live and on-demand fitness classes. The app also uses AI to customize your workout recommendations based on past performances. Apps such as Peloton provide a glimpse of how fitness will evolve in the near future, in which personalization is the king. These AI-powered platforms go beyond routine workouts and can tailor suggestions to meet your goals, fitness level, and performance.
6. Centr
Who doesn’t know about ‘Centr”? It has a broad selection of workout routines that include cardio, strength training, and yoga. It also employs AI to tailor your workouts based on your objectives and preferences. The Centr offers a wide range of varied workouts, including HIIT and strength training, yoga, and MMA.
7. Sworkit
Some people need access to expensive equipment. Sworkit, a free application with premium features, uses AI to offer efficient bodyweight exercises that you can complete anywhere and at any time. The app tailors bodyweight workout routines according to your fitness level and creates custom routines to suit your requirements. This is ideal for people who want to exercise at home or cannot access the gym.
8. Future
Future has an app for personal trainers that analyzes your weaknesses and develops an exercise program specifically tailored to tackle them. The app is subscription-based for fitness and uses AI to achieve precisely this. Therefore, it’s not a mistake to assert that the future is more than just tracking your performance. Its powerful AI analyzes your workout data and pinpoints areas where you could be in a lag.
9. Hevy
Hevy, a fitness app, uses AI to transform your phone into the perfect fitness partner. Whether you’re a veteran bodybuilder or a fitness novice, Hevy empowers you to reach your goals by offering features that allow you to record your exercises, track progress, and effortlessly achieve your fitness goals. AI creates custom workout routines that are tailored to your requirements and the equipment you have available.
Also Read : 7 Lifestyle Apps That’ll Make Your Life Flawless
How can you Create an AI application?
This step-by-step guide will show you how a fitness app development company construct and utilize an AI application. Whether you’re an entrepreneur, researcher, or simply interested in AI technological advancements, these steps can help you understand the process of AI app development that will revolutionize your business.
1. Problem Identification and Setting Goals
First, determine the problem that must be resolved before creating an AI application. Consider the processes and functions of the app you would like to employ to implement the AI technologies. What results can you expect from it? What will you gain from it? After you’ve identified the issue and the idea, it is time to create requirements for your product. Based on the requirements analysis, mobile app development company in UAE can comprehend the reason behind creating products and discover ways to assist them.
Additionally, you will need to complete the following tasks at the time of planning:
Choose the composition of technical and non-technical teams – from business analysts and project managers to data engineers and backend developers.
Discuss your plan with a professional.
2. Begin Exploring the Data Needed to Create an AI/ML Model
To ensure an AI program’s success, it’s essential to begin by setting clearly defined goals; inspirational speaker Tony Robbins eloquently says, “Setting goals is the first step in shifting the invisible into the visible.” The goals you set will act as the guiding light for your development, keeping the process in focus and aligning with your goals, ensuring everyone is on the same level.
The key to the best AI app is having a clear idea of its objectives right from the beginning. Start by identifying the exact issue your application aims to address to benefit its customers. For example, think about Grammarly, which aims to improve user writing by providing real-time corrections of style and grammar, which it can achieve.
When your main goal has been established, you must define KPIs. Key Performance Indicators (KPIs) adhere to the SMART (Specific, Measurable, Relevant, time-framed) framework for assessing the app’s performance. The AI-specific KPIs would include:
- Model accuracy
- Model precision
- Model recall
- F1-score
- Model fairness
These metrics are crucial to assessing the effectiveness of your AI model. In addition, establishing precise milestones for deployment and development is vital, and using an agile development process such as Scrum will help facilitate this process.
Before you begin the process of developing, review the resources available to you. AI models are heavy on resources and will require extensive infrastructure that can support both the maintenance and training phases. Be sure that your infrastructure is sturdy enough to meet the demands.
Ultimately, the objectives you set at the start of the AI app development will guide you through the entire process, highlighting the importance of correcting them at the beginning.
Also Read : How does an Artificial Intelligence App add Great Potential to your Business?
3. Preparation of Data
The preparation and collection of information are the basis of the development of AI applications. Even the most advanced AI models’ performance depends on the high quality and accuracy of the data they’re trained with. It is crucial to prioritize data quality over quantity to reduce the significant costs associated with data that is not good. The results of a Gartner study revealed that companies anticipate losses of up to $15 million per year because of poor data quality.
To prevent such losses, it is vital to ensure that your data is cleansed and formatted. Making the best choice of data sets to train AI models is an initial step. For those who are working with natural language processing models, Common Crawl offers a large, free database of data from web crawls, which was used for 82 percent of the tokens that were raw used in the GPT-3 model developed by OpenAI.
Platforms such as Kaggle or AWS Data Exchange also provide numerous datasets that can be used without cost. After selecting your datasets, the following steps require thorough data preparation through cleaning, processing, and wrangling.
Cleaning data eliminates errors like duplicate entries using tools like OpenRefine and Tibco Clarity.
Preprocessing can include transformation, integration smoothing, normalization, and reduction. Each step improves the accuracy of the data.
Data wrangling transforms the processed dataset into one appropriate for use in training AI models. Following these steps, your data is prepared to be used in modeling training or improving the model’s performance.
Effective data collection and preparation aren’t just the initial steps but are crucial in the development of a solid AI application. It’s not just about making it happen but doing it properly.
Also Read : Enjoy Weight Loss with a Personalized Weight Loss App
4. Choosing the Right Tools and Frameworks
The performance of your AI application is dependent on choosing the appropriate frameworks and tools to create and train your models. Your choice should align with your particular business requirements and seamlessly integrate with your current infrastructure. Choosing the right tools will speed up the development process and boost the efficiency of your AI models.
Let’s look at some of the most popular AI frameworks that currently dominate the field:
TensorFlow is a flexible open-source library ideal for creating and deploying machine-learning models. It is adept at managing complex projects and excels for commercial uses, specifically in tasks requiring image recognition.
For example, Airbnb leveraged TensorFlow to create an AI-driven image classification system to help improve the classification of listings photos. Furthermore, TensorFlow offers an integrated platform called TensorFlow Extended (TFX), which allows the management of all your AI models from training to deployment.
PyTorch is well-known for its modular and flexible design, which makes it a popular choice for researchers due to its simplicity in use in experimental environments. However, it is specifically designed for use in academia and does not have commercial support, which could be a disadvantage for certain enterprise applications.
Google AutoML stands out as an excellent choice for teams new to AI. It lets users train custom models quickly with no technical knowledge, which makes it perfect for applications that require less technical skills.
Outside of these models, there are a variety of cloud-based AI platforms that provide complete environments for training, developing, and using AI models:
- Google Cloud AI
- Amazon SageMaker
- Azure Machine Learning
They are provided by the top cloud-based service companies, which implies that you’re already using one. This can dramatically ease the integration and implementation of the AI models.
The essence is that choosing the appropriate tool to accomplish the task is crucial. The success of your AI app’s performance depends on this choice, which underscores the importance of strategically selecting the right AI development frameworks and tools.
Training and Design of AI Models.
The design phase starts with defining the structure of your model based on the problem it intends to solve. This is how you can proceed:
1. Choose the Training Approach
- Supervised learning: This is best for situations where the outcomes are known, and data is labeled. It’s widely employed in applications that require high accuracy, like weather forecasting and facial recognition.
- Unsupervised Learning: This method can uncover hidden patterns or intricate patterns within data, such as clustering for anomaly detection tasks.
- Reinforcement Learning: It is highly effective for decision-making processes. The model learns how to behave in a given environment by performing specific actions and gaining rewards. This approach is particularly beneficial in areas like gaming and robotics.
Also Read : A Parental Control App: Modern Solutions for Managing Children’s Online Safety
2. Select the Right Model Architecture
- Convolutional Neural Networks (CNNs): CNNs are primarily used for video and image recognition. However, CNNs can also be used in every pattern recognition algorithm because they can precisely recognize features regardless of the image’s noise and distortions.
- Recurrent Neural Networks (RNNs): RNNs are appropriate for processing sequenced data, such as time series analysis or natural language processing, where the order of data points is essential.
- Generative Adversarial Networks (GANs): GANs are ideal for creating new data based on input data. They are commonly used to create realistic images, video enhancement, and art.
3. Training preparation
- Data Splitting: Separate Data into three components: training, validation, and testing. The most common split is 70% for training to enable the model to acquire as much knowledge as possible, 15% for validation to fine-tune the parameters, and 15% for testing to assess the model’s performance.
- Features Engineering: This involves modifying, selecting, or creating new features using the raw data to enhance the ability of the model to detect patterns.
4. The Training of Your AI Model
- Feeding data: The model is trained using the data from your training. This process involves multiple iterations in which the model predicts and makes changes to improve.
- Hyperparameter Tuning: Modify the model’s settings (hyperparameters) in the verification process to determine the most optimal configuration to achieve the highest performance.
- Cross-validation: Employ cross-validation methods during training to test the model’s effectiveness across various dataset parts.
5. Evaluating and Integrating the Model
- Testing: After training your model, test it with the test data that you have not seen. This is essential to ensure that the model works in real-world situations.
- KPI measurement: Examine the model’s performance using predetermined KPIs, such as accuracy recall, precision, F1 score, and the ROC-AUC, based on the app’s requirements.
Also Read : When E-commerce Meets AI: Explore The Perfect Match for Fashion
6. Innovative Approaches to Generative AI
Advanced methods such as embedding retrieval-augmented Generation (RAG) and fine-tuning using generative AI applications can be applied. These are essential to increasing the capabilities and performance of AI models. Let’s take an analysis of how these methods can be implemented:
7. Embeddings in Generative AI
Embeddings are a vital component of numerous AI applications, specifically Generative AI. They convert high-dimensional data (like images or text) into a smaller space while maintaining semantic relationships. This involves presenting data elements (such as phrases, words, or words) as vectors within a unidirectional vector space where semantically related items are closer. This method is vital in tasks like text generation that require understanding and maintaining context.
8. Retrieval-Augmented Generation (RAG)
RAG is a complex method that combines the effectiveness of generative models and Information retrieval technology. This method is a hybrid approach that enhances the capacity of models to provide precise and relevant responses to context through:
- Retrieving Information: Once an inquiry is received, the information retrieval mechanism uses it to pull relevant information from a huge data set, also known as knowledge bases.
- Enhancing Generation: obtained information is fed into a generative model incorporating this context to create unison and contextually enhanced response.
This method is beneficial for chatbots and question-answering systems, where providing exact and accurate responses is essential.
9. Fine-Tuning
Fine-tuning is the process by which an already-trained model is developed (or “fine-tuned”) on an entirely new, usually smaller and smaller, data set that is specialized to a specific area or task. This technique uses the general abilities of the model derived from a massive dataset and then adjusts it to the particulars of a specific task. The steps involved in fine-tuning are:
- Prepared Data: The data is ready but usually needs preprocessing steps like normalization or tokenization. Labeling is also a possibility.
- Adjustment of the Model: The trained model’s parameters are slightly altered to reflect the updated data, making it more efficient in completing this new job.
- Training Model: It is taught based on the latest data, with a focus on improving instead of creating a new model. This usually uses fewer computational resources and takes less time than learning an entire model from scratch.
Fine-tuning improves models’ performance in specific tasks, significantly improving their accuracy and relevance to the application’s requirements.
With these new techniques, fitness app development company can develop more robust, flexible, and effective Generative AI models adapted to the requirements of their specific applications.
10. Model Integration in the App
Incorporating an AI algorithm into your application is a crucial step in the development process. It’s the most important factor in an AI application’s performance. This crucial step determines whether your application’s AI capabilities will improve user experience or not meet expectations.
The first choice you’ll have to make is whether to integrate your AI model into the application’s front end or the back end. AI models are often incorporated into the front end to interact in real-time with customers. However, some models, like voice recognition models, may be more effective when included in the backend to improve accuracy and processing power.
Consider the next step: the place where the AI algorithm will be processing the data either in the cloud or on your device. Processing on-device, also known as edge AI, is especially beneficial in AI-powered Internet of Things (IoT) applications offering greater flexibility and security. However, cloud-based processing is generally more effective in most cases because of its capacity and capacity.
Integration of AI model cannot be simple. It’s unnecessary to start from scratch when AI APIs already satisfy your requirements. For example, Google Cloud Vision API has extensive AI imaging capabilities that can be seamlessly integrated into your application, saving development time.
Furthermore, it’s crucial to create a feedback loop in your application. This feature lets users give feedback and evaluate their experience with the AI model, providing vital information that can be used to improve and refine the model continually.
Transparency regarding your application’s AI capabilities is also crucial. Being transparent about how AI operates and the advantages it brings can help build confidence with your customers. Despite the complexity of the AI model, user interactions should be easy.
The bottom line is that creating an AI application is about more than just integrating technology. It’s about ensuring this technology will significantly improve the overall experience for the app users.
Also Read : How Much Does it Cost to Create a Mobile App in Dubai in 2024?
11. Model Testing and Iteration
The creation of your AI application isn’t just a one-time moment; it’s the beginning of a continuous process of refinement and enhancement. Considering each AI design as a continuous development process, constant testing and refinement are required to ensure growth and improvements.
AI models could be better at the time of their creation. They need to be taught from experience and evolve to change over time. In addition, regular retraining with up-to-date data is vital to stop model drift, a common issue. The model’s performance decreases due to changes in data patterns. Continuous updating keeps the model current and up-to-date throughout its lifespan.
What methods can you use to test your AI application successfully? There are three types of testing you should concentrate on:
Tests for unit functionality: This step examines the most minor components of your app separately. For example, if your app uses an AI chatbot, it is possible to test whether its greeting feature works correctly. Automating these tests allows for constant checks and does not burden the QA engineers.
The integrity testing step examines the extent to which your application’s different components interact. If you’re developing an AI-driven app, it is crucial to determine how your AI models interact with the application’s other components under circumstances that simulate real-world situations. This ensures that the application is working smoothly as a unit.
User Acceptance Testing (UAT) is the final phase of testing. It assures that your application matches the requirements and expectations of its users. It is beneficial to include an array of users during the testing phase to collect a wide range of feedback. This will give valuable insights into user preferences and behavior.
In addition to these basic types of testing and methods, the QA engineers may also be involved in various other testing methods during the fitness app development process. However, integration, unit, and user acceptance testing are essential to ensuring the success of an AI application.
Integrating feedback loops is a must. It lets you capture and continuously integrate feedback from users, allowing for iterative improvements that are in line with the users’ demands and boost the efficiency of your application.
If you adopt this rigorous test framework and keep an open channel for user feedback, you can ensure that your AI app is efficient and relevant for the foreseeable future.
Also Read : How much does it cost to develop an on-demand app?
Cost to Develop a Personal Trainer App Like Aaptiv
The cost to develop a mobile app that resembles Aaptiv ranges from $10,000 to $40,000. However, it’s important to be aware that the cost is fluctuating, meaning that many variables and components could be considered in the final price.
Before we go further, let’s take an overview of the Aaptiv app cost:
App Development- $10,000 to $20,000
Creation of content- $5,000 to $10,000
Design (UI/UX)- $2,000 to $5,000
Project Management- $2,000 to $4,000
Complexity – $1,000 to $5,000
NOTE: Understand that this is merely an estimation. Costs can differ, so contact the appropriate mobile app development company in Saudi Arabia to find the best price.
Conclusion
In conclusion, the expense of creating an AI trainer app like Aaptiv has many facets. Collaboration with an AI app development company, specifically one skilled in AI chatbot development, is crucial.
While considering your options, consider the importance of employing mobile app development in Dubai and investing in a smooth user interface. Suppose you prioritize these aspects and focus on them. In that case, you’ll be well on establishing an innovative AI personal trainer application that stands out in a competitive fitness technology landscape.
Techugo, with its expertise in AI and mobile app development, can be your ideal partner in this endeavor. Our team of skilled developers has a proven track record of creating successful fitness apps. We understand the intricacies of building an AI-powered personal trainer and can guide you through the entire development process. From concept to launch, Techugo provides comprehensive support, ensuring your app is not just functional but also engaging and user-friendly.
Let’s work together to create a fitness revolution! Get in touch with us today to discuss how you want your AI trainer app like Aaptiv to be.
Get in touch.
Write Us
sales@techugo.comOr fill this form